Conventionally, control of computing devices has been done through manual interaction, mostly in the form of using a mouse and keyboard. As technology evolves we observe additional ways of interacting with the machine, i.e. motion sensors, speech recognition, eye tracking etc, enhancing the capabilities and experience of the user. The next step to this evolution are Brain Computer Interfaces (BCIs).
BCIs allow the machine to directly capture the mental activity of the user. This is accomplished by equipping the user with sensors specifically designed for capturing changes in neural activity. Such a device is the Electroencephalograph (EEG). EEGs use electrodes to monitor potential differences between different areas of the human scalp, capturing electrical discharges that are created during the activation of the subject’s neurons. EEGs have great temporal resolution due to sampling rates above 1000 Hz and high spatial resolution of 5-9 cm [1]. Additionally, non-invasive EEGs are also portable, easy and safe to use devices, allowing the public to take advantage of them in a variety of applications.
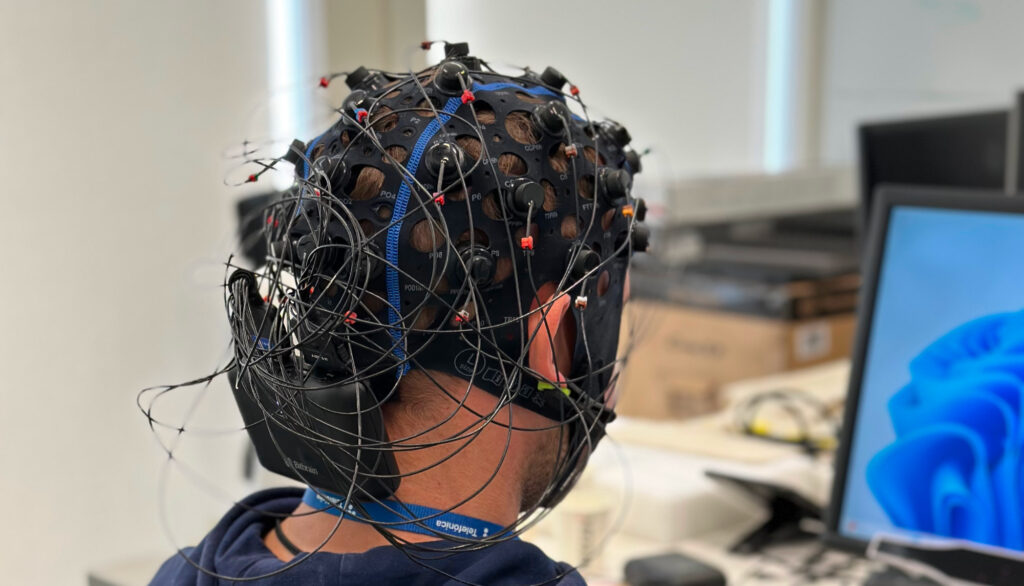
The usage of EEG based BCIs is promising but also challenging. Although rapid in the capture of neural activity, the signal is sensitive to external factors. A vast amount of noise is mixed with the useful signal. This noise originates from muscle movement, electrode detachment and ambient electrical current [2]. Moreover the signal’s nature is itself very complex. Responses to stimuli can differ from user to user but also from state to state.
Although the aforementioned facts make thought decomposition a daunting task, numerous applications have been able to predict and take advantage of the user’s cognitive state. Using modern algorithms in the form of convolution and transformer-based networks, researchers have been able to classify EEG signals with high accuracy, in some cases reaching up to 90% [3,4]. The signals detected are referred to as Event Related Potentials, or ERPs. ERPs are changes in the brain function caused due to external stimuli. It is interesting to note that in certain works the authors report being able to classify ERP related EEG signals while using minimally pre-processed data [4,5].
In our case, the InfoVis system will take advantage of these accurate transformer based architectures, giving it a more robust estimation of the users fatigue and stress levels. In turn, the InfoVis system will be able to properly adapt the user interface based on the particular situation, improving its usability. With advancements in consumer-level EEG headsets, such technologies will be able to provide those advantages to the common folk. In summary, EEG enhanced InfoVis systems hold the promise of revolutionising user-friendly interfaces and we are excited to lead our work in that direction.