We are thrilled to share that our abstract, titled “Adaptive Visualization Framework for Human-Centric Data Interaction in Time-Critical Environments,” has been accepted for presentation at the 11th International Conference on Human Interaction & Emerging Technologies (IHIET-AI 2024). This work is a significant downstate of the importance of the SYMBIOTIK project goals towards making data visualization more accessible and intuitive, especially in time-sensitive contexts such as Security Operation Centers and domain-specific monitoring dashboards.
The challenge
In today’s data-driven era, the task of making objective decisions through complex information visualization systems is increasingly daunting. This is particularly true when humans must navigate information overload, understand data clearly, and interact efficiently with machines. The challenge is even more significant for non-expert human operators, as thinking in purely numerical and mathematical abstractions can be unnatural, especially in critical situations.
The solution
The abstract presents the SYMBIOTIK approach to tackle this problem by developing self-adapting visualizations tailored to the user’s cognitive level, which evolve alongside their increasing expertise and learn from past experiences. The proposed framework integrates biological principles, collaborative and social-like properties, and tailor-made agents guided by AI techniques for context awareness, emotion sensing, and expressing capabilities to achieve this. In addition, the proposed framework incorporates a mechanism to deliver self-adapting visualizations tailored to the user’s cognitive level, evolving in response to their growing expertise and learning from previous experiences. The system enables continuous learning and integration capabilities through knowledge exchange and analysis loops that are formulated via data communication pipelines across the operational environment, the processing components, and the visualization interface.
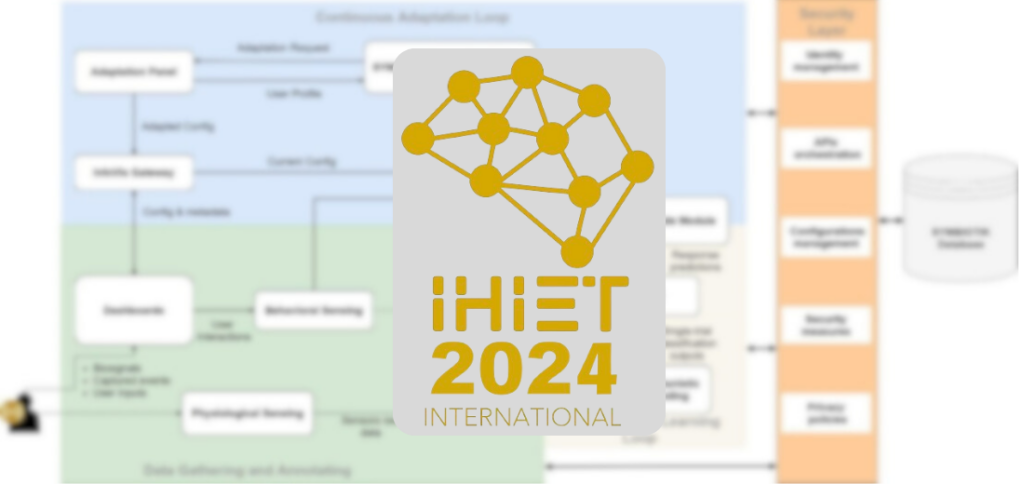
The proposed high-level architecture consists of four main layers: i) data gathering and annotating; ii) Reinforcement Learning Loop; iii) Continuous Adaptation Loop and iv) Security Layer. The framework is empowered with cross-modal sensors to collect multimodal behavioral signals that will be analyzed using various signal processing and ML algorithms. In addition, custom event listeners and dashboard usability captors are employed to collect user preferences and feedback and identify visualization trends and activity patterns based on the analysis of usage statistics. All this information will be used to feed real-time data ingestion techniques combined with ML-driven neural-mechanistic understanding mechanisms and data representation and transformation capabilities that are developed to create a robust and comprehensive workflow toward the adaptation policy.
We are excited about the potential impact of our work and look forward to sharing our findings at the conference. Stay tuned for more updates!