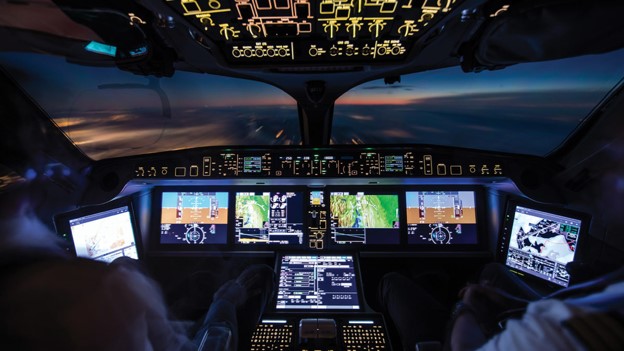
Automation is at the heart of most technologies we use in our daily life, with applications ranging from automotive (e.g., manual vs. automatic transmission system and autopilots) and household appliances (e.g., kettle, washing machine) to industrial machinery and healthcare systems (e.g., patient monitoring, autonomous surgery). Reducing the need for human intervention in automated processes brings forth several advantages, such as the reduction in labor cost and relief from routine operations, reduction in material and energy costs, as well as increase in industrial production and improvement in organizational efficiency [1]–[3]. However, reduction of human intervention also means that the operator (user) of an automated system is relegated to a monitoring role, losing this way his/her task proficiency over time. Furthermore, operators often over-rely on the system and, thus, pay less attention to the task-relevant environment. As a result, the operators can have a limited situation awareness and, in case of automation fail, they could face the challenge of initiating proper recovery actions. In several cases, such as with cockpit automation, impaired perception of the situation due to high levels of automation has led to tragic accidents [4].
In response to these concerns, several researchers have proposed the use of adaptive automation, which refers to intelligent systems in which the task control is distributed between a user and a machine, to ensure that the cognitive and emotional state of the user is kept within desired levels. This distribution can vary depending on the context of use, which covers parameters known for the task, the end user, the platform and devices used, and the environment in which the user is working.
Self-rating and behavioral tests can be used to assess the user state. However, investigators typically prefer Brain-Computer Interfaces (BCIs), which are a powerful framework for bridging the gap between the brain and an external device. By integrating sensors that measure brain activity with real-time processing algorithms, and sometimes in conjunction with additional physiological signals (e.g., heart rate, pupil dilation, electrodermal activity), passive BCIs can provide a continuous and objective assessment of user state in an unobtrusive manner (i.e. without interrupting the operator from the task) [5]. Aspects of user state that are commonly examined are mental workload, situation awareness, attention levels and fatigue.
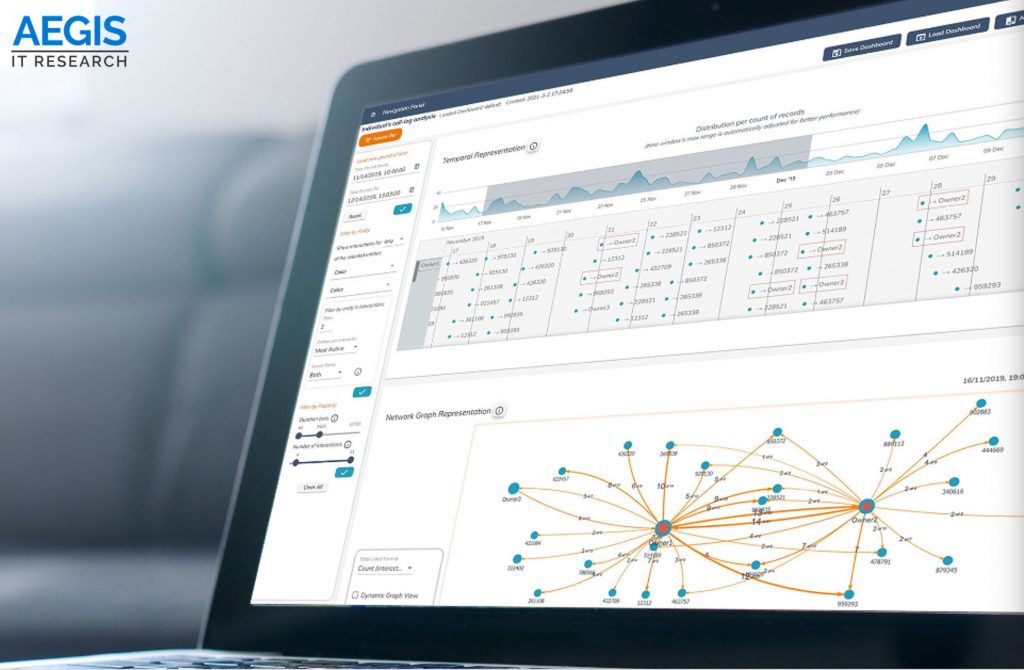
BCIs have been used for several complex tasks, such as air-traffic control [6] and flight-related tasks [7], to control the level of automation. These systems were found to mitigate the users’ mental workload while also improving their performance (e.g., reducing flight time). In addition, BCIs have been developed for preventing information overload in operators in industrial production lines as well as everyday tasks. For instance, systems that can detect a driver’s mental workload based on EEG measures have been used for controlling the volume of information the driver is exposed to, which has been shown to decrease the drivers’ reaction time [8]. Finally, adaptive automation based on BCI has also been proposed for entertainment-related purposes, such as for personalizing the experience of museum visitors based on their emotional state [9] or for controlling the functions of a character in a game [10].
Despite the extensive research in adaptive automation, we are only scratching the surface of BCI applications, partly because existing algorithms do not handle well the large dimensionality of physiological signals. At SYMBIOTIK, we are exploring new directions for analyzing these signals (electroencephalography, functional near-infrared spectroscopy, eye gaze, heart rate, etc.) by leveraging Artificial Intelligence (AI). Particularly, we are interested in developing AI-based BCI solutions for improving interaction between human and information visualization (InfoVis) systems. The outcome of this project has the potential to facilitate decision making in different contexts of use such as with critical cases in the emergency room or with investigations for preventing terrorist attacks.
[1] M. Yip and N. Das, “Robot autonomy for surgery,” in The Encyclopedia of Medical Robotics, 2019, pp. 281–313. doi: 10.1142/9789813232266_0010.
[2] K. Jha, A. Doshi, P. Patel, and M. Shah, “A comprehensive review on automation in agriculture using artificial intelligence,” Artif. Intell. Agric., vol. 2, pp. 1–12, Jun. 2019, doi: 10.1016/j.aiia.2019.05.004.
[3] M. G. Ippolito, E. Riva Sanseverino, and G. Zizzo, “Impact of building automation control systems and technical building management systems on the energy performance class of residential buildings: An Italian case study,” Energy Build., vol. 69, pp. 33–40, Feb. 2014, doi: 10.1016/j.enbuild.2013.10.025.
[4] N. B. Sarter and D. D. Woods, “‘From Tool to Agent’: The Evolution of (Cockpit) Automation and Its Impact on Human-Machine Coordination,” Proc. Hum. Factors Ergon. Soc. Annu. Meet., vol. 39, no. 1, pp. 79–83, Oct. 1995, doi: 10.1177/154193129503900119.
[5] T. O. Zander, J. Brönstrup, R. Lorenz, and L. R. Krol, “Towards BCI-Based Implicit Control in Human–Computer Interaction,” in Advances in Physiological Computing, S. H. Fairclough and K. Gilleade, Eds. London: Springer London, 2014, pp. 67–90. doi: 10.1007/978-1-4471-6392-3_4.
[6] P. Aricò et al., “Adaptive Automation Triggered by EEG-Based Mental Workload Index: A Passive Brain-Computer Interface Application in Realistic Air Traffic Control Environment,” Front. Hum. Neurosci., vol. 10, Oct. 2016, doi: 10.3389/fnhum.2016.00539.
[7] L. J. Prinzel, F. G. Freeman, M. W. Scerbo, P. J. Mikulka, and A. T. Pope, “A Closed-Loop System for Examining Psychophysiological Measures for Adaptive Task Allocation,” Int. J. Aviat. Psychol., vol. 10, no. 4, pp. 393–410, Oct. 2000, doi: 10.1207/S15327108IJAP1004_6.
[8] G. Dornhege, J. del R. Millán, T. Hinterberger, D. J. McFarland, and K.-R. Müller, Eds., “24 Improving Human Performance in a Real Operating Environment through Real-Time Mental Workload Detection,” in Toward Brain-Computer Interfacing, The MIT Press, 2007. doi: 10.7551/mitpress/7493.003.0031.
[9] Y. Abdelrahman, M. Hassib, M. G. Marquez, M. Funk, and A. Schmidt, “Implicit Engagement Detection for Interactive Museums Using Brain-Computer Interfaces,” in Proceedings of the 17th International Conference on Human-Computer Interaction with Mobile Devices and Services Adjunct, Copenhagen Denmark, Aug. 2015, pp. 838–845. doi: 10.1145/2786567.2793709.
[10] B. van de Laar, H. Gurkok, D. Plass-Oude Bos, M. Poel, and A. Nijholt, “Experiencing BCI Control in a Popular Computer Game,” IEEE Trans. Comput. Intell. AI Games, vol. 5, no. 2, pp. 176–184, Jun. 2013, doi: 10.1109/TCIAIG.2013.2253778.