Brain Computer Interfaces (BCIs) may revolutionize the way humans interact with computers by establishing a direct communication pathway with our brains. At the core of these interfaces there is signal processing, which plays a pivotal role in extracting valuable information from brain activity. In this post, we delve into the fascinating world of signal processing in BCI technology.
Numerous algorithms have been developed to process brain signals, especially electroencephalogram (EEG) data, encompassing a wide range of operations, such as time-domain analysis, frequency-domain analysis, spatial-domain analysis, and multiway processing [1]. In general, EEG signal is considered the output of a nonstationary nonlinear system, primarily influenced by changes in brain metabolism. Modeling this system remains complex and unresolved. However, the nonstationarity of EEG signals can be quantified by measuring certain statistics at different time lags. If these statistics show minimal variation, then the signals can be considered stationary. While the multichannel EEG distribution is generally assumed to be multivariate gaussian, it is important to note that the mean and covariance properties often vary from one segment to another. As a result, EEGs are considered to be stationary only within short intervals, known as quasistationarity. Several instances of nonstationarity in EEG signals can be observed, such as changes in alertness and wakefulness, characterized by stronger alpha oscillations, eye blinking, transitions between different ictal states, and in event-related potential (ERP) and evoked potential (EP) signals [1, 2].
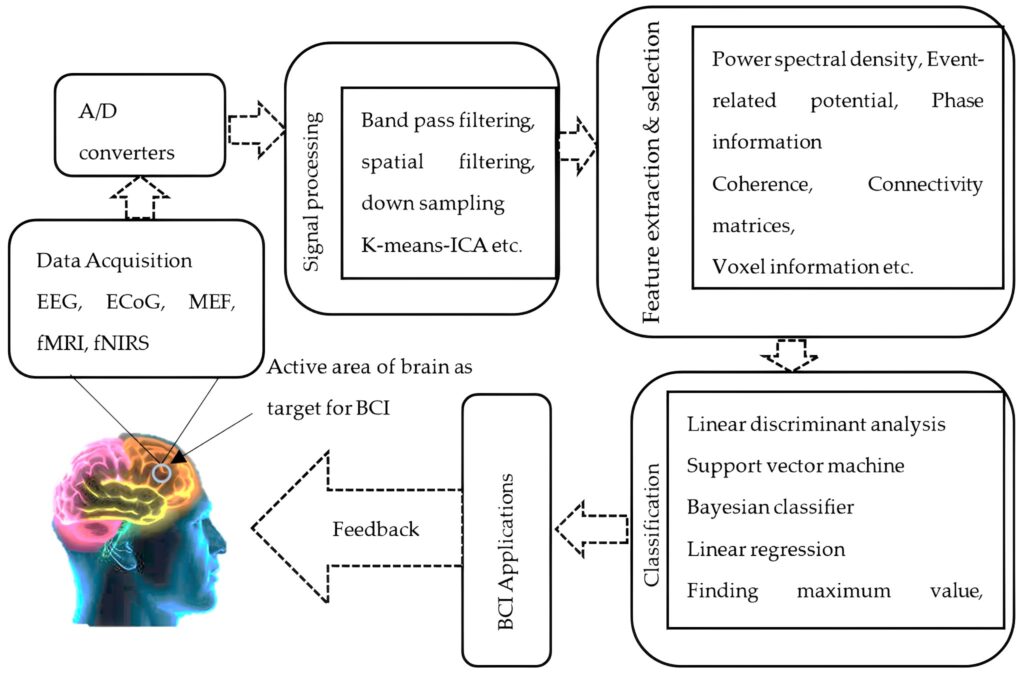
The measurement of signal segment distribution change can be carried out in terms of both the parameters of a gaussian process and the deviation of the distribution from gaussian. Non-Gaussianity of the signals can be examined by measuring or estimating higher-order moments like skewness, kurtosis, negentropy, and Kulback–Leibler (KL) divergence. In the following we describe some specific signal processing methods commonly used in BCI.
Common Spatial Pattern (CSP) identifies spatial filters that maximize the variance between various classes of EEG signals (e.g., negative vs. positive emotions), which usually improves classification performance [3]. Fast Fourier Transform (FFT) with windowing techniques transforms the time-domain EEG signal into the frequency domain, providing information about the power distribution across different frequency bands. Common Average Reference (CAR) is a method to improve EEG data quality by reducing common noise across electrodes [1, 2]. Independent Component Analysis (ICA) is a blind source separation technique used to split EEG signals into independent components, which helps to identify and remove artifacts [4]. Autoregressive Models estimate the power spectral density of EEG signals as a linear combination of past samples. Hidden Markov Models (HMM) are probabilistic models that can be used to model the temporal dynamics of brain signals, particularly in tasks where users produce sequential brain patterns [5]. Finally, wavelet transform is an alternative time-frequency analysis technique that provides information about frequency components over time, making it suitable for transient brain responses [6].
To conclude, these signal processing methods play a crucial role in decoding brain signals and enabling the interaction between humans and computers through BCI devices. They contribute to improving the accuracy, efficiency, and usability of BCIs, leading to advancements in various applications, including assistive technology, neurorehabilitation, and brain-controlled devices.
References
- Sanei, S., Chambers, J. A. (2013). EEG Signal Processing. Germany: Wiley, ISBN 978-0-470-02581-9
- Rajesh, Rao., Reinhold, Scherer. (2010). Statistical Signal Processing for Neuroscience and Neurotechnology, Academic Press, ISBN 9780123750273
- Basar, M. D., Duru, A. D., & Akan, A. (2020). Emotional state detection based on common spatial patterns of EEG. Signal, Image and Video Processing, 14(3), 473–481. doi:10.1007/s11760-019-01580-8
- Wang, G., Teng, C., Li, K., Zhang, Z., & Yan, X. (2016). The Removal of EOG Artifacts From EEG Signals Using Independent Component Analysis and Multivariate Empirical Mode Decomposition. IEEE Journal of Biomedical and Health Informatics, 20(5), 1301-1308
- Williams, N. J., Daly, I., & Nasuto, S. J. (2018). Markov Model-Based Method to Analyze Time-Varying Networks in EEG Task-Related Data. Frontiers in Computational Neuroscience, 12. doi:10.3389/fncom.2018.00076
- Almanza-Conejo, O., Almanza-Ojeda, D. L., Contreras-Hernandez, J. L., & Ibarra-Manzano, M. A. (2023). Emotion recognition in EEG signals using the continuous wavelet transform and CNNs. Neural Computing and Applications, 35(2), 1409–1422. doi:10.1007/s00521-022-07843-9