Recent advancements in Artificial Intelligence (AI), particularly in Natural Language Processing (NLP) and Computer Vision (CV), have brought us closer to emulating and even surpassing human capabilities. The development of transformer neural architectures like chatGPT and latent diffusion models like MidJourney has had a profound impact on the field. The rapid progress of AI has far-reaching societal consequences, with new industries emerging at an unprecedented rate and significant implications for the job market.1,2
Notably, prominent figures in the field have expressed concerns and called for regulation. Geoff Hinton, a pioneer of deep learning, left Google3 to have the freedom to criticize AI safely. Gary Marcus, known for advocating symbolic AI over deep learning, initially wrote an essay titled “Deep learning is hitting a wall,”4 only to later support an open letter,5 signed by over 27,000 scientists, urging a pause on large-scale AI experiments. Even rivals like Hinton and Marcus6 have put aside their differences to acknowledge the need for AI regulation.7 This shift is reflected in discussions held by Sam Altman (CEO of OpenAI), Gary Marcus, and Christina Montgomery (IBM) at the US Senate8 on how to regulate AI for improved safety.9
The convergence of AI, neuroscience, and psychology has become a topic of great interest.10 While intelligence is closely tied to the workings of the animal brain, the influence of neuroscience on computational theory has often been overlooked. Some researchers argue that neuroscience has lost relevance in shaping the AI field, despite its historical importance. On the other hand, there are AI manifestos that neglect the contributions of psychology and neuroscience. The relationship between these fields seems to be a game of musical chairs, with AI researchers sometimes overlooking the valuable insights from neuroscience and psychology.
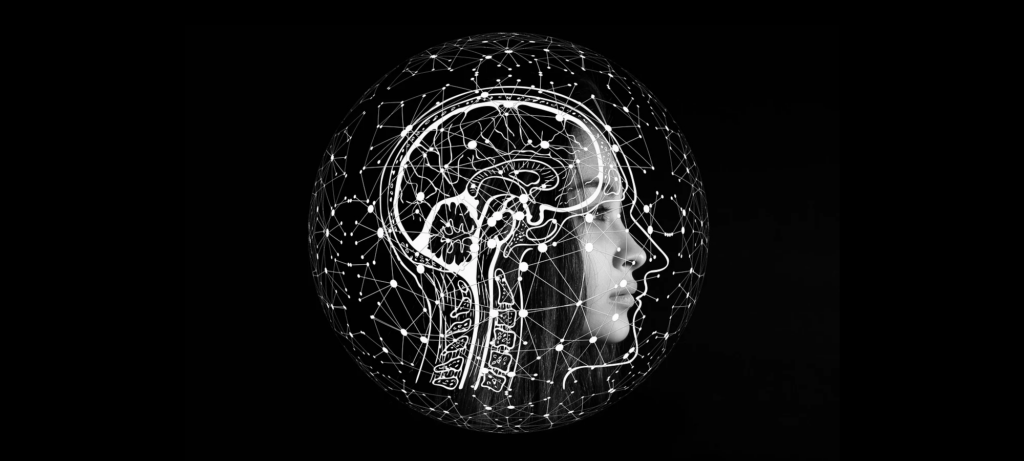
One argument supporting the integration of AI and biology is that algorithms optimized for solving engineering problems often serve as successful models of brain function. Although biologists did not invent technical concepts solely based on neurobiological discoveries, the theoretical arsenal came from other domains, independent of neurobiology. For example, Convolutional Neural Networks (CNNs) were inspired by studies of the visual system but made assumptions that contradicted biological data. These biologically implausible assumptions, such as shift invariance, turned out to be practically important, as they allowed weight sharing among convolutional filters, reducing the number of parameters to be learned. Similar arguments apply to reinforcement learning, suggesting that future synergies between AI and biology hold promise.
The exploration of parameter space in AI also aligns with the understanding of degeneracy, where dissimilar neural architectures and parameters can yield similar results. It is akin to finding that different objects, like a toaster and an iron, can both make toast—they serve the same function. Researchers studying degeneracy,11 such as Prof. Dr. Eve Marder,12 conduct trial-and-error explorations of parameter value combinations to mimic biological behavior. This approach helps uncover how distinct circuit properties and parameters in different brains and their corresponding AI implementations can still exhibit identical behaviors. Another example of the interconnectedness of AI, neuroscience, and psychology is the “self-attention” mechanism in transformer architectures. Prof. Dr. Alex Graves,13 a renowned expert in deep learning, has described attention14 as an introspective or internal process that allows us to focus on one thought or event at a time by intentionally ignoring other information. The implementation details of attention mechanisms mark a divergence between AI and psychology.
In summary, the recent advancements in AI, particularly in NLP and CV, have propelled us toward emulating human capabilities. The integration of AI, neuroscience, and psychology holds great potential for future advancements, with researchers recognizing the importance of each field’s contributions. The convergence of these disciplines promises exciting synergies and a deeper understanding of intelligence and behavior.
References
- https://arxiv.org/abs/2303.101302
- https://papers.ssrn.com/sol3/papers.cfm?abstract_id=4375268
- https://www.nytimes.com/2023/05/01/technology/ai-google-chatbot-engineer-quits-hinton.html
- https://nautil.us/deep-learning-is-hitting-a-wall-238440/
- https://futureoflife.org/open-letter/pause-giant-ai-experiments/
- https://twitter.com/GaryMarcus/status/1070868222105112576
- https://garymarcus.substack.com/p/ai-risks-short-term-and-longand-what
- https://www.nytimes.com/2023/05/16/technology/openai-altman-artificial-intelligence-regulation.html
- https://twitter.com/sama/status/1511735572880011272
- https://www.oneusefulthing.org/p/becoming-strange-in-the-long-singularity
- https://www.ncbi.nlm.nih.gov/pmc/articles/PMC5123929/
- https://www.nature.com/articles/nn1352
- https://arxiv.org/abs/1308.0850
- https://youtu.be/AIiwuClvH6k